What Separates Good and Bad Data
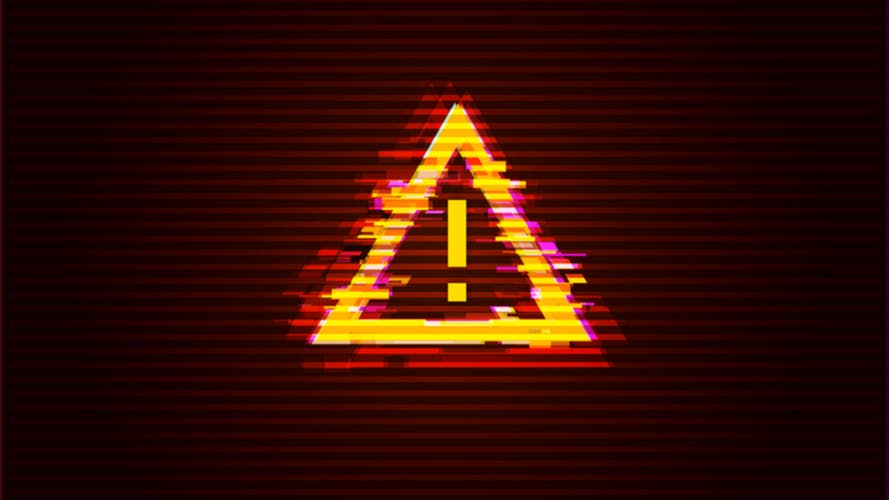
No matter what industry you’re in, chances are that you’ve been told about the importance of data analytics in some capacity. Data lets you learn more insight about your company performance, guides your future decisions, and gives you more information about your competitors.
What many people neglect to mention is these benefits only apply to good data. Bad data is arguably more damaging to a company than having no data, so it’s important you know what can render data unusable. Here are some key signs your data is problematic:
Inaccuracy: In many cases, bad data is built off of information that is either incomplete or flat-out wrong. It’s important that if you’re basing a decision off of internal data, that you see how comprehensive said data is. For example, if you want a look at a company’s financial history for trends, but you only have 2 years out of a 10-year span, that data can present a very inaccurate look at your finances. You could be ignoring entire trends that make a huge difference for your future. In addition to missing data, you also want to make sure the data you have wasn’t gathered with biased or outdated methods.
Poorly applied data: In some cases, your statistics are correct, but you use them incorrectly. This tends to happen a lot with industry novices who don’t understand what impact a certain metric has on their overall business. For example, in the CPG sector, it’s easy for companies to get hung up on how many signups they get to their loyalty programs. However, are these customers truly loyal ones or savings-minded ones who use loyalty discounts for every company they buy from? Not knowing the difference could paint an inaccurate picture.
Not checking your data vendors: In many cases, small businesses want to reap the benefits of a data-based format, but don’t have the funds or manpower to do it all alone. This means bringing on a third party or using software to help extract data or insights. There’s nothing necessarily wrong with this, but you need to do your due diligence on who you decide to work with. What types of methods are they using? Can they supply references from other satisfied customers? Putting your data in the hands of another company is a major point of importance. Make sure you choose wisely.
Human error: Finally, we need to address possibly the largest contributor to bad data issues — human error. You can do everything else right, from your sources to your planning, but one mis-entered number in your data spreadsheets could spoil everything. The only real check here you have is being thorough. To give you an idea, as much as 80% of the work that professional data scientists do is cleaning the data before going into any insights.
In many cases, you need an expert hand to correct the systemic issues that lead to bad data. Reach out to the Modus team for more analysis, insight, and support.